The median is a statistical measure that represents the middle value of a dataset when the data is assorted in numerical order. Unlike the mean, which is sensitive to outliers, the median is a more robust measure of central tendency. This makes it particularly useful for analyzing data that may contain extreme values.
To find the median, first, arrange the data in ascending or descending order. If the data set contains an odd number of values, the median is the middle value. If the data set contains an even number of values, the median is the average of the two middle values.
For example, if we have the following data set: {1, 3, 5, 7, 9}, the median is 5. This is because 5 is the middle value when the data set is assorted in ascending order. If we have the following data set: {1, 3, 5, 7, 9, 11}, the median is 6. This is because the two middle values are 5 and 7, and their average is 6.
The median is a useful statistic for a variety of reasons. It is a simple and straightforward measure to calculate, and it is not affected by outliers. Additionally, the median can be used to compare data sets of different sizes.
How to Find the Median
The median is a statistical measure that represents the middle value of a dataset when assorted in numerical order. It is a robust measure of central tendency, not affected by outliers, making it useful for analyzing data that may contain extreme values.
- Definition: The middle value of a dataset assorted in numerical order.
- Importance: A robust measure of central tendency, unaffected by outliers.
- Calculation: Arrange the data in ascending or descending order and identify the middle value (for odd datasets) or the average of the two middle values (for even datasets).
- Example: In the dataset {1, 3, 5, 7, 9}, the median is 5.
- Comparison: The median is less sensitive to outliers compared to the mean.
- Applications: Useful for comparing data sets of different sizes, analyzing skewed data, and identifying trends.
- Advantages: Simple to calculate, not affected by outliers, provides a clear representation of central tendency.
- Disadvantages: Can be less informative for small datasets, may not accurately represent the entire dataset if there are extreme values.
These aspects collectively provide a comprehensive understanding of how to find the median, its significance, and its applications. The median serves as a valuable statistical tool for analyzing and interpreting data, offering a robust measure of central tendency that is particularly useful when dealing with skewed data or outliers.
Definition
This definition forms the cornerstone of understanding how to find the median. The median is a statistical measure that represents the middle value of a dataset when assorted in numerical order. It is a robust measure of central tendency, not affected by outliers, making it useful for analyzing data that may contain extreme values.
-
Facet 1: Identifying the Middle Value
To find the median, we need to identify the middle value of the dataset. For an odd number of values, the median is the middle value. For an even number of values, the median is the average of the two middle values.
-
Facet 2: Numerical Ordering
Before identifying the middle value, we need to ensure that the dataset is assorted in numerical order. This involves arranging the data points from smallest to largest or vice versa.
-
Facet 3: Dealing with Outliers
The median is not affected by outliers, which are extreme values that can skew the mean. This makes the median a more reliable measure of central tendency when the data contains outliers.
-
Facet 4: Comparison to Mean
The median differs from the mean, which is another measure of central tendency. The mean is calculated by adding all the values in a dataset and dividing by the number of values. Unlike the median, the mean can be affected by outliers.
By understanding these facets, we gain a comprehensive view of how the definition of the median guides us in finding the median value of a dataset. This involves identifying the middle value, ensuring numerical ordering, considering outliers, and comparing it to the mean.
Importance
The importance of the median as a robust measure of central tendency, unaffected by outliers, is deeply connected to how we find the median. Outliers are extreme values that can significantly distort the mean, another measure of central tendency. However, the median remains stable even in the presence of outliers, making it a more reliable representation of the typical value in a dataset.
For instance, consider a dataset representing the incomes of a group of individuals. If one individual earns a significantly higher income than the rest, the mean income will be inflated, giving a misleading impression of the typical income level. In contrast, the median income will not be affected by this outlier, providing a more accurate representation of the central tendency.
Understanding this connection is crucial because it guides us in choosing the appropriate measure of central tendency for our analysis. When dealing with data that may contain outliers, the median is the preferred choice as it provides a more robust and reliable representation of the typical value.
Calculation
The calculation of the median involves arranging the data in ascending or descending order and identifying the middle value for odd datasets or the average of the two middle values for even datasets. This step is a fundamental component of “how to find the median” as it allows us to determine the central value of the dataset.
To illustrate, consider the dataset {1, 3, 5, 7, 9}. Arranging the data in ascending order, we get {1, 3, 5, 7, 9}. Since the dataset contains an odd number of values, the middle value is 5, which is the median. In contrast, for the dataset {1, 3, 5, 7, 9, 11}, arranging the data in ascending order gives {1, 3, 5, 7, 9, 11}. Since the dataset contains an even number of values, the median is the average of the two middle values, which is (7+9)/2 = 8.
Understanding this calculation is crucial because it provides a systematic approach to finding the median. By arranging the data in numerical order and identifying the middle value or the average of the two middle values, we can efficiently determine the central tendency of the dataset, which is essential for statistical analysis and interpretation.
Example
This example is a concrete illustration of the process of finding the median, which is a fundamental concept in statistics. The dataset {1, 3, 5, 7, 9} represents a set of numerical values, and the median is a measure of central tendency that indicates the middle value when the data is assorted in numerical order. In this case, we can see that the data is already assorted in ascending order, which makes it straightforward to identify the median.
The median is important because it provides a stable and reliable representation of the central tendency of a dataset, especially when the data may contain outliers or extreme values. Unlike the mean, which is sensitive to outliers, the median is not affected by these extreme values, making it a more robust measure. Therefore, understanding how to find the median is essential for.
In practice, finding the median is a common task in various fields such as research, data analysis, and statistics. It is used to compare datasets, identify trends, and make informed decisions based on the central tendency of the data. By understanding how to find the median, we gain a valuable tool for understanding and interpreting numerical data.
Comparison
The comparison between the median and the mean regarding their sensitivity to outliers is a crucial aspect of understanding how to find the median. Outliers are extreme values that can significantly distort the mean, leading to a misleading representation of the central tendency of a dataset. In contrast, the median remains stable even in the presence of outliers, making it a more robust measure.
To illustrate this concept, consider the following example. Suppose we have a dataset representing the test scores of a group of students: {90, 95, 98, 100, 105}. The mean score is 97.6, which is heavily influenced by the outlier value of 105. The median score, however, is 98, which is not affected by the outlier and provides a more accurate representation of the typical score.
Understanding this comparison is essential because it guides us in choosing the appropriate measure of central tendency for our analysis. When dealing with data that may contain outliers, the median is the preferred choice as it is less sensitive to extreme values and provides a more reliable representation of the typical value.
In practice, this understanding is particularly important in fields such as finance, healthcare, and research, where outliers can significantly impact the interpretation of data. By considering the sensitivity to outliers when finding the median, we can make more informed decisions based on the central tendency of the data.
Applications
Understanding how to find the median is crucial for various applications, including comparing data sets of different sizes, analyzing skewed data, and identifying trends. These applications highlight the versatility and importance of the median as a statistical measure.
-
Comparing Data Sets of Different Sizes
The median allows for the comparison of data sets with different sample sizes. Unlike the mean, which can be affected by the number of data points, the median remains a stable measure of central tendency, enabling meaningful comparisons across data sets of varying sizes.
-
Analyzing Skewed Data
The median is particularly useful for analyzing skewed data, where the distribution of data points is not symmetrical. In such cases, the median provides a more accurate representation of the central tendency compared to the mean, which can be heavily influenced by extreme values.
-
Identifying Trends
The median can be used to identify trends in data over time or across different groups. By calculating the median for different subsets of data, it is possible to observe changes or differences in the central tendency, helping to uncover patterns and trends.
These applications demonstrate the practical significance of finding the median. By understanding the role of the median in these contexts, we can effectively utilize this statistical measure to gain valuable insights from data analysis.
Advantages
The advantages of the median lie in its simplicity, robustness against outliers, and ability to provide a clear representation of central tendency. These attributes are closely intertwined with the process of finding the median.
-
Simplicity of Calculation
Finding the median involves arranging the data in numerical order and identifying the middle value. This straightforward calculation makes it accessible and easy to apply, even for large datasets.
-
Resistance to Outliers
The median’s insensitivity to outliers stems from its reliance on the middle value. Outliers, which can significantly distort the mean, have minimal impact on the median, making it a more stable measure for data with extreme values.
-
Clear Representation of Central Tendency
The median provides a clear and intuitive representation of the central tendency of a dataset. Unlike the mean, which can be influenced by extreme values, the median offers a more representative value that aligns with our understanding of “middle” or “average”.
These advantages collectively contribute to the utility of the median in various applications. Its simplicity allows for efficient calculation, its resistance to outliers ensures reliability in data analysis, and its clear representation of central tendency aids in effective interpretation and decision-making.
Disadvantages
Understanding the disadvantages of the median, namely its potential limitations with small datasets and extreme values, is crucial in the context of “how to find the median.” These disadvantages stem from the inherent characteristics of the median and its calculation.
-
Less Informative for Small Datasets
The median can be less informative when dealing with small datasets. In such cases, the median may not fully capture the variability and distribution of the data, potentially leading to a less representative understanding of the central tendency.
-
Inaccurate Representation with Extreme Values
While the median is generally robust against outliers, it may not accurately represent the entire dataset if there are extreme values. Extreme values can disproportionately influence the median, especially in small datasets, resulting in a skewed representation of the central tendency.
These disadvantages highlight the importance of considering the size of the dataset and the presence of extreme values when interpreting the median. In situations where these factors may affect the reliability of the median, alternative measures of central tendency, such as the mean or mode, should be considered.
Frequently Asked Questions
This section addresses some common concerns or misconceptions regarding how to find the median.
Question 1: Is the median always the middle value in a dataset?
Not necessarily. For odd-sized datasets, the median is indeed the middle value. However, for even-sized datasets, the median is the average of the two middle values.
Question 2: How is the median affected by outliers?
The median is relatively unaffected by outliers, unlike the mean. Outliers are extreme values that can skew the mean away from the center of the data. However, the median remains stable, providing a more robust measure of central tendency.
Question 3: When is it appropriate to use the median instead of the mean?
The median is preferred over the mean when the data contains outliers or when the distribution of data is skewed. In such cases, the median provides a more accurate representation of the central tendency.
Question 4: How can I find the median of a large dataset efficiently?
For large datasets, it may not be practical to manually sort the data. Instead, statistical software or programming libraries can be used to calculate the median efficiently.
Question 5: What is the difference between the median and the mode?
The median is the middle value of a dataset, while the mode is the value that occurs most frequently. The median is a measure of central tendency, while the mode is a measure of commonality.
Question 6: How is the median used in real-world applications?
The median has numerous applications, including comparing datasets, analyzing skewed data, identifying trends, and making informed decisions based on the central tendency of data.
These FAQs provide a deeper understanding of how to find the median and its practical implications. By addressing common concerns and misconceptions, we aim to enhance your knowledge and ability to effectively utilize the median in data analysis.
Transition to the next article section…
Tips for Finding the Median
Calculating the median effectively requires a systematic approach. Here are several tips to guide you:
Tip 1: Arrange the Data
Before finding the median, arrange the data in ascending or descending order. This organization simplifies the identification of the middle value or the average of the two middle values.
Tip 2: Identify the Middle Value
For odd-sized datasets, the median is the middle value after arranging the data numerically. For even-sized datasets, the median is the average of the two middle values.
Tip 3: Consider Outliers
Outliers are extreme values that may distort the mean. However, the median remains unaffected by outliers, providing a more robust measure of central tendency.
Tip 4: Use Statistical Tools
For large datasets, manual calculation can be tedious. Statistical software or programming libraries offer efficient methods to find the median.
Tip 5: Interpret the Median
The median provides valuable insights into the central tendency of the data. It is particularly useful when dealing with skewed data or outliers.
Summary: Understanding how to find the median empowers data analysts and researchers to accurately represent and interpret central tendency. By following these tips, you can effectively calculate the median and gain meaningful insights from your data.
Transition to the Conclusion: The median plays a crucial role in statistical analysis, offering a robust and reliable measure of central tendency. These tips provide a practical guide to finding the median, enabling you to confidently apply this valuable statistical tool in your research and data analysis endeavors.
Conclusion
Finding the median is a fundamental statistical technique used to determine the central tendency of a dataset, particularly when dealing with skewed data or outliers. Understanding how to find the median empowers researchers and analysts to accurately represent and interpret their data.
This article has explored the concept of the median, its advantages and disadvantages, and practical tips for its calculation. By mastering this statistical tool, individuals can effectively analyze data, draw meaningful conclusions, and make informed decisions based on the central tendency of their datasets.
Youtube Video:
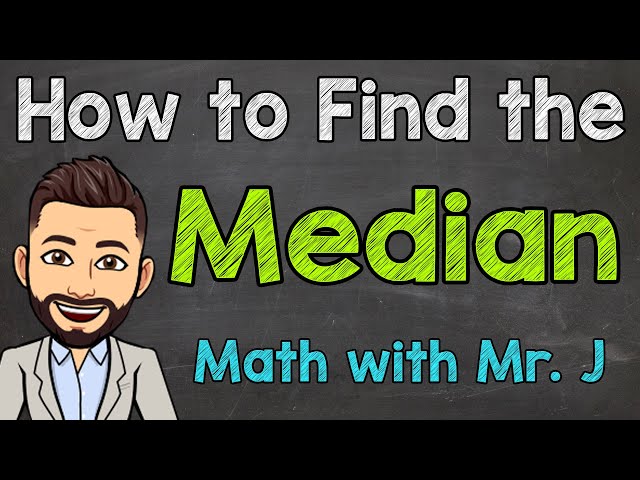